Earth System Science graduate student helping define the future of climate change modeling
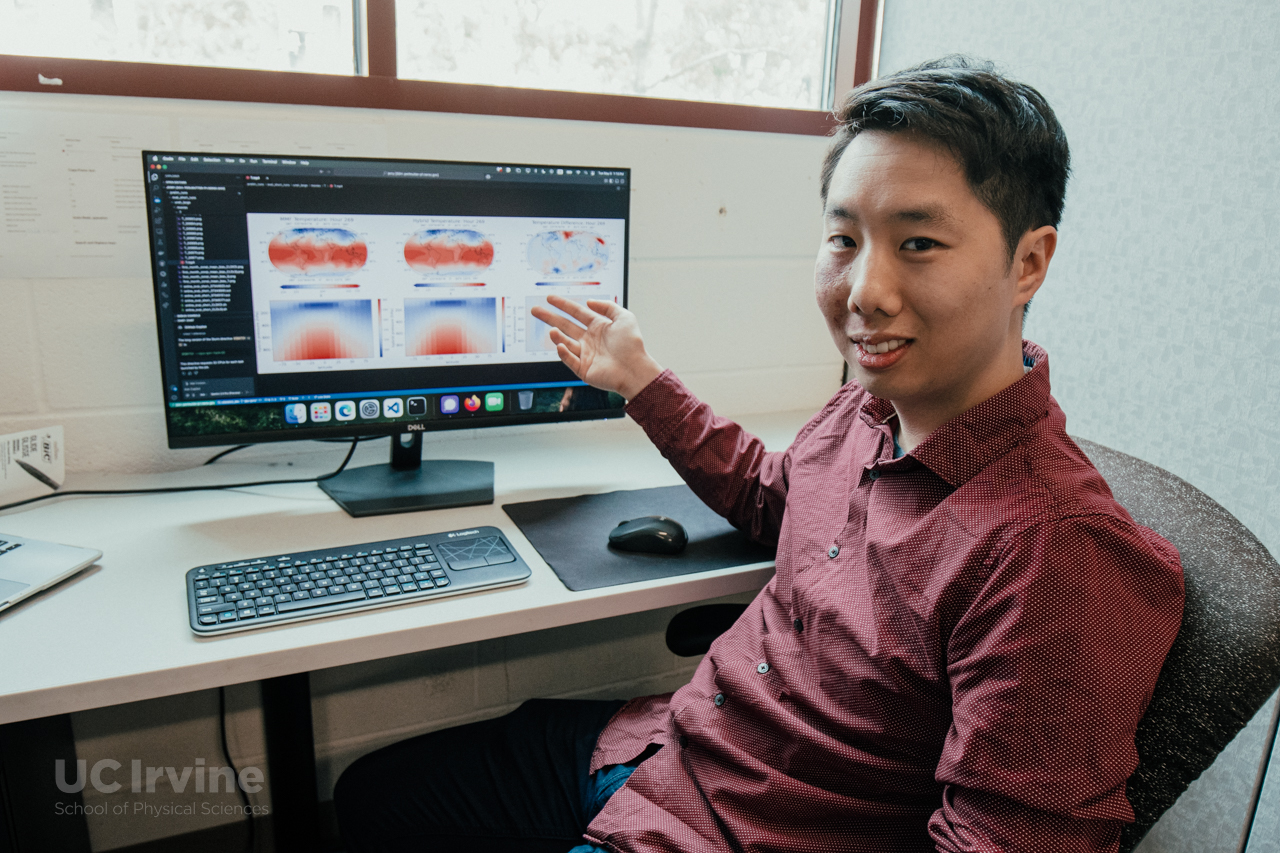
UC Irvine Earth System Science graduate student Jerry Lin explains how AI is transforming climate modeling research, something he recently helped others learn about by co-running a workshop in Vienna. "I definitely would not have been able to pull off organizing that workshop on my own," said Lin, "It was only made possible by support from LEAP, a NSF Science and Technology Center I am fortunate to be funded by, as well as various connections I have made over the years."
Artificial intelligence stands to revolutionize the way climate scientists design and run climate model simulations by allowing researchers to better model detailed physical dynamics in Earth’s climate system. In a recent Journal of Advances in Modeling Earth Systems (JAMES) study, a team led by Jerry Lin – a Ph.D. candidate in the UC Irvine Department of Earth System Science – and Professor Michael Pritchard tackle an issue standing in the way of reliable AI-enhanced climate models: the performance of machine learning (ML) algorithms can be unpredictable when researchers use them to run hybrid physics-ML climate simulations..
The UC Irvine School of Physical Sciences caught up with Lin to hear more about the research, and to learn about how he’s helping other climate scientists integrate AI-related methods into their own work, be it through co-organizing a Machine Learning for Earth System Modeling (ML4ESM) workshop at International Conference on Machine Learning (ICML) in Vienna, Austria or running a Kaggle competition to help stimulate machine learning-climate physics research.
“Jerry’s work is at the leading edge of this field," said Pritchard. "This is tough, technical work that took years of software engineering. I expect it to have a long tail of impact."
SoPS: So, what does your JAMES paper help reveal about machine learning and climate change models?
JL: This paper helps us narrow down how we need to go about designing hybrid physics-machine learning climate models. For a while, researchers in my domain were frustrated by the fact that a machine learning emulator could yield great results on a dataset but unexpectedly perform poorly when dynamically evaluated inside a climate model. My paper samples the coupled behavior of these emulators at scale (think thousands of climate simulations) to statistically assess what design decisions actually matter for getting hybrid physics-machine learning climate models to work reliably.
SoPS: What inspires you to do this kind of work?
JL: I have always been worried about climate change ever since I first learned about it, but I was also fascinated by advances in machine learning. Before applying to grad school, I had some intuition that machine learning methods could thrive in the big data environment of climate science, and learning about my advisor's research concretely showcased a few ways those methods could revolutionize climate modeling. That convinced me to apply to his group, and I consider myself exceptionally lucky to have been given this opportunity.
SoPS: Tell me about the machine learning workshop you ran in Vienna. Who was the audience, and how did it go?
JL: I conceived of and co-organized a Machine Learning for Earth System Modeling workshop at the ICML. The audience consisted of machine learning researchers looking to apply their latest advances to grand challenges in climate science. We invited speakers from a range of domains to expose these researchers to different kinds of problems in our domain. We had many incredible submissions to our workshop from researchers I truly admire and awesome presentations from our invited speakers. I definitely would not have been able to pull off organizing this workshop on my own, and it was only made possible by support from LEAP – an NSF Science and Technology Center I am fortunate to be funded by, as well as various connections I have made over the years.
SoPS: What came of that AI climate Kaggle competition you ran?
JL: The competition helped expose our community to breakthrough neural network architectures and design decisions that we had never even heard of. These are architectures that were only invented in the past few years and are just now starting to be tested inside hybrid physics-ML climate models. Additionally, other innovations that showed up in the winning submissions, like using a "confidence loss" to predict the model's own loss on a given input, have been quietly adopted by many researchers all around the world because of its amazing benefits and ease-of-use.
SoPS: What’s next for you research-wise?
JL: My immediate research involves integrating winning architectures and ideas from the competition into hybrid physics-machine learning versions of an earth system model called E3SM-MMF. After I defend this summer, I plan to start a postdoc at Boston University working under Professor Elizabeth A. Barnes, working on using interpretability tools in machine learning for climate research.