UCI researchers develop algorithm to help predict the final size of a wildfire
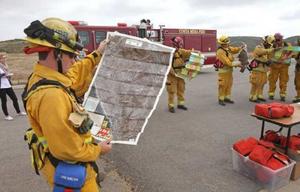
A Newport Beach firefighter examines a map in 2011 that outlined information about hydrants and other factors vital to fighting a wildfire similar to the Laguna Beach firestorm of 1993. A machine learning model developed by UCI researchers could help determine the final size of a wildfire.
It’s kind of like predicting whether a social media post will go viral. Only in this case, the stakes could be much higher.
A team of researchers at UC Irvine says it has developed a machine learning model based on a “decision tree” algorithm that — if given information on climate data, atmospheric conditions and the types of vegetation present — can help determine the final size of a wildfire, starting from the moment of ignition.
“Our approach was to learn through machine learning” — the use of a computer system to identify patterns in data sets — “and identify the important variables and what combinations of those variables are good predictors of final fire size,” said Shane Coffield, a UCI doctoral student in earth science and lead author of the study.
A useful analogy to thinking about the elements that could factor into a fire’s total size is figuring out what could make a post on social media go viral, Coffield said.
“Some small fraction of posts or tweets will end up going viral,” he said. “You could ask, ‘What are some of the factors that might control that?’ If Beyoncé tweeted something vs. I do, the percent of users probably matters, and how diverse and connected users are. We think about [virality] too as scientists.”
“What research can we do that will be really impactful?” he added. “We think about the network of fuels around the ignition and other variables like weather. We’re trying to identify just with the information that we have whether it’s going to be a big fire or not.”
The study was supported by UCI’s Machine Learning and Physical Sciences program and the National Science Foundation.
Researchers began work in fall 2017, selecting Alaska as the study site for the machine learning model because the state has been affected by several wildfires in its boreal forests in the past decade, which Coffield said is largely driven by the effects of climate change.
Factors making it especially vulnerable to fire are increasingly longer, hotter summers and lightning strikes. Another is the vegetation in those forests — particularly black spruce trees.
“Black spruce, which are dominant in Alaska, have these long, droopy branches that are designed — from an evolutionary perspective — to wick up fire,” said co-author James Randerson, a professor in earth system science at UCI. “Their seeds are adapted to do well in a post-fire environment, so their strategy is to kill off everything else around them during a fire to reduce competition for their offspring.”
In 2015, the Los Angeles Times reported that Alaskan fire officials historically didn’t intervene with wildfires because of the state’s vastness and sparse population. Now, Coffield said, “it’s getting to the point where entire ecosystems are getting damaged by those fires and the smoke is starting to hurt people.”
Researchers determined that the two main predictors for wildfires in Alaska were the vapor pressure deficit — basically, a measurement of how dry the air is — and whether there were enough black spruce trees within a 2½-mile radius of the starting point. The model’s projections worked about 50% of the time, researchers said.
“In California, you could probably apply the same model, but the variables would be different. The [vapor pressure deficit] would still be a factor, but probably also the temperature and wind,” Coffield said. “That trifecta could really lead fires to get out of control in a really short period of time.”
The data provided by the algorithm, he said, could help scientists studying wildfires but also, because of its relative simplicity, help fire managers on the ground allocate resources to contain the blazes.
The algorithm operates with relative ease and speed. It “learns” with each new data point and can calculate the critical thresholds for identifying large fires. In the long run, that can be beneficial in cases of multiple, simultaneous fires, Coffield said.
Researchers are now investigating the possibility of predicting fire growth day by day and are turning their attention to a broad look at California.
“It’s a collaboration with other [University of California campuses] and we’ll be trying to understand how climate is driving the fires that we’ve been seeing [in the state], how to predict them and maybe what the future of California will look like in terms of fires, droughts and ecosystem health,” Coffield said.
The team is in its first year of the new study, which is projected to last three years.